Conjoint Analysis to Optimize B2B SaaS Pricing
- Troy Wendt
- Mar 18
- 9 min read
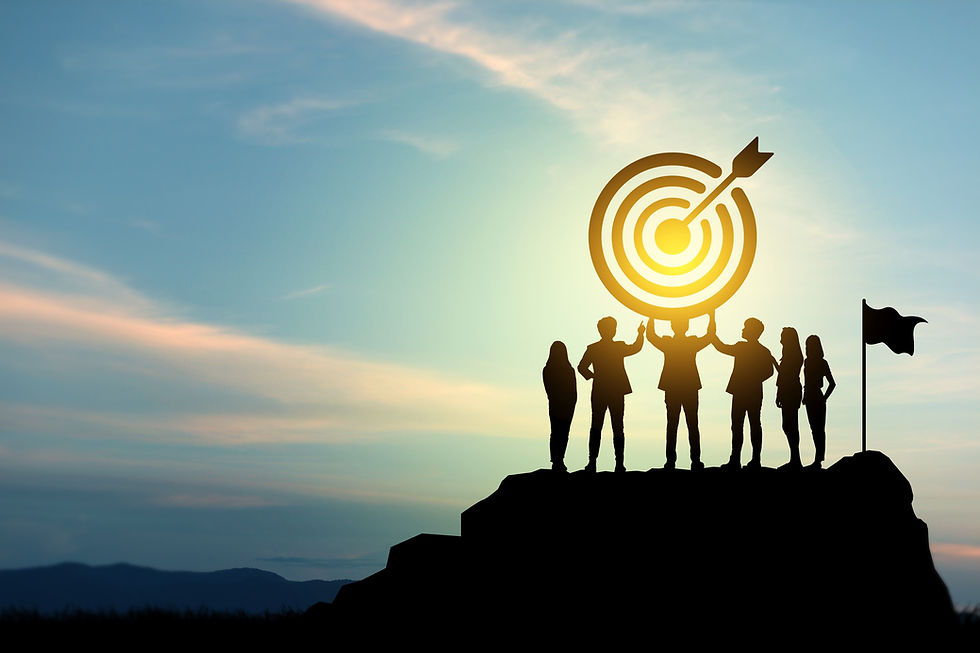
Optimizing pricing and packaging is one of the most powerful levers for driving growth in B2B SaaS. Improvements in pricing can create immense value. Let’s put some numbers on that. Alex Clayton of Spark Capital did a valuation study of publicly traded SaaS companies. The analysis yielded a formula for SaaS company valuation which is 29 x ARR x annual growth rate. This suggests mathematically that valuation is proportional to both ARR and growth rate. If optimized pricing for a $200M valuation SaaS company increases ARR by 10% and growth rate by $5%, the valuation of the company increases by $31M. Depending on the current ARR of your company, optimized pricing can easily create millions or tens of millions of dollars in value.
According to Megan Peitz, CEO of market research firm Numerious, “conjoint analysis is ideal for B2B SaaS because it enables you to decode the preferences of the buyer, optimize pricing and packaging, and maximize profits". Although conjoint analysis is complex and relatively expensive, it is an extraordinary tool to get high-stakes decisions right.
We’ll begin our journey into conjoint analysis by reviewing your available options to conduct market research for pricing.
Options for Pricing Market Research
There are three standard market research techniques that can be used to optimize pricing: Van Westendorp, Gabor-Granger, and conjoint analysis. We’ll briefly review each technique.
Van Westendorp
The Van Westendorp Price Sensitivity Meter asks the respondent four questions for a single product:
At what price would you consider the product to be so expensive that you would not consider buying it? (Too expensive)
At what price would you consider the product to be priced so low that you would feel the quality couldn’t be very good? (Too cheap)
At what price would you consider the product starting to get expensive, so that it is not out of the question, but you would have to give some thought to buying it? (Expensive/High Side)
At what price would you consider the product to be a bargain—a great buy for the money? (Cheap/Good Value) (Source: Wikipedia)
The Van Westendorp technique then plots cumulative distribution curves for the responses to each of these four questions. The optimal price point is defined by the intersection of the “expensive” and “inexpensive” curves. This point represents the price at which a balanced number of respondents consider the price to be getting expensive versus being a good bargain. Van Westendorp is a useful technique for a new, simple product without a well-defined existing market.
Gabor–Granger
The Gabor–Granger method estimates optimal pricing using questions about predefined price points. In a Gabor-Granger survey respondents are presented with a series of prices for one product—often starting at a baseline price and then moving up and down in a ladder format. For each price point, they are asked whether they would purchase the product at that price. If they accept the price, they are asked about a higher price. If they reject the price, they are asked about a lower price. This helps pinpoint the maximum price each survey participant is willing to pay.
This willingness to pay data is then used to estimate the ideal price point. For each respondent, the Gabor-Granger variable is the highest price they are willing to pay. The collection of Gabor-Granger variables is utilized to build a demand curve and find the revenue optimizing price.
Conjoint Analysis
Conjoint analysis helps companies understand how customers value different features, services and pricing levels. In B2B SaaS, companies typically have a number of product features and support options that are priced in different configurations. With conjoint, each respondent is modeled to calculate their total value of any combination of features and price. By predicting product value for each survey respondent, you can calculate the market share of hypothetical products for both your company and competitors across various scenarios. This ability to calculate the share of product choice for a wide range of configurations and prices is used to optimize feature bundling and price levels.
Conjoint analysis has two distinct advantages over Van Westendorp and Gabor-Granger. First, in conjoint, any combination of features and price points can be analyzed to optimize pricing. In contrast, Van Westendrop and Gabor-Granger can only be used to test a single product configuration. Second, among the three techniques only conjoint analysis can account for competitive products in the market. A detailed description of conjoint analysis follows.
A Deeper Look at Conjoint Analysis
Conjoint analysis is a discrete choice market research technique where survey participants choose the best option from multiple hypothetical products. The methodology simulates real-world choice among competing products.
Two key aspects of conjoint analysis with specific definitions are attributes and levels. An attribute is a product feature or characteristic. Levels are the versions of each attribute to be tested. Each attribute in a conjoint study must have two or more levels. An attribute can have any number of levels, but in typical practice has 2-7 levels.
An instructive product we’ll use to illustrate conjoint analysis is mobile phones which have distinct attributes like brand, screen size, battery life, memory, camera quality, and price. The survey participant chooses their preferred option from different phone configurations of these attributes. Here is an example of cell phone attributes and levels:
Brand (Apple, Samsung, Google)
Screen size (6 inch, 7 inch)
Battery life (10 hours, 20 hours, 30 hours)
Memory (128 GB, 256 GB, 512 GB)
Camera (Good, Professional)
Price ($599, $799, $999)
An sample conjoint survey question for mobile phones is below:
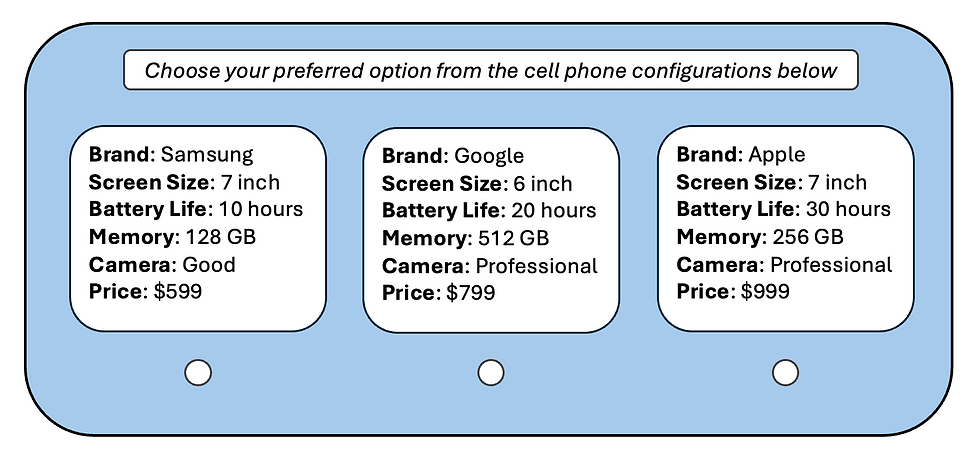
If you were a participant in a conjoint survey you would answer something like 15 of these “choice tasks” where you select the best option from the product choices presented. Often the number of product choices range from 3-5. The magic of conjoint is that based on the respondent’s choices, the value of every level of every attribute can be calculated. These values are called utilities or part worths. An amazing feature of conjoint analysis is that the part worths can then be added (conjoined) for any combination of attribute levels to calculate the total worth of that product configuration for each participant. This enables a choice prediction for each respondent between products with any combination of attributes and levels. The product with the highest sum of part worths wins the choice contest.
Here is an illustration of what the part worths might look like for a participant in our mobile phone survey:

Two typical characteristics of part worths are that as features improve, part worths rise and as price goes up, part worths drop. Better features have higher utility, and higher price has lower utility. One level of each attribute is chosen to specify a product, and the total value of the product is the sum of part worths. With the ability to estimate product values for each survey participant, it is possible to build a market simulator with specialized software or a spreadsheet. With a market simulator you can specify hypothetical products for your company as well as competitive products, and the simulator will calculate “share of choice”. Share of choice is the percentage that each product in the simulation is the top choice among research participants.
Here is an example of share of choice calculations for 5 mobile phone configurations:

Pricing Prep Work for a B2B SaaS Conjoint Study
B2B SaaS differs from consumer products like cell phones and televisions in that there are an array of pricing models and pricing metrics that can be used. Whereas a television has a single price per TV with attributes including screen size, picture quality, etc, a B2B SaaS product could have usage-based pricing or per-seat pricing, and features can be configured into a wide range of product offerings. Due to the varied characteristics of B2B SaaS pricing models, I recommend pre-work using a structured pricing process to choose a top candidate pricing model before the conjoint analysis is done. In particular, it is very helpful to choose the principal pricing metric prior to the conjoint. A single pricing metric allows for more accurate measurement of feature value. Further, all of the key features to be utilized in the pricing model should be identified prior to the conjoint.
Designing and Executing the Survey
A key aspect of survey design is choosing the attributes and levels to be studied in the conjoint. Attributes include product features and support options. It is better to choose only the key features as attributes to improve the accuracy of survey results. 7 to 10 attributes is an ideal limit to allow research participants to consider each attribute individually and not use simplification strategies that reduce the quality of results. The levels for each attribute are also needed to design the survey.
The range of prices to be studied should be specified prior to survey design. The price range is for hypothetical products that include all attributes. The prices are used to construct the product options presented to research participants. Either discrete prices or a continuous price range can be used.
With attributes, levels and prices fully defined, the next step in the process is survey design. An experimental design called fractional factorial design is often used. The objective of experiment design is to achieve a balance of attribute levels to be studied, and minimize the number of hypothetical products while capturing the necessary data. The survey design should be implemented in the conjoint software of choice. Leading vendors of conjoint software include Sawtooth Software, Qualtrics and Conjointly.
The participants for your survey must be identified. A research panel is often used, but another approach is to email the survey to potential participants from your customer and prospect database. A research panel is usually selected using a firm that specializes in sourcing research participants. If your company has important segmentation considered in your pricing strategy, the panel can be selected with equal distribution by segment.
The next step is to distribute the survey to your target audience. Throughout the data collection phase survey quality and response rate should be monitored. The survey typically continues until the target number of valid responses is reached.
Optimizing Pricing and Packaging
With the survey complete, the conjoint outputs can be prepared to optimize pricing and packaging. The three most useful outputs to optimize pricing are attribute importance, willingness to pay, and the market simulator.
Attribute importance quantifies how much each attribute influences overall decision making. It is calculated by comparing the range of part worths, and is expressed as a percentage. Attribute importance ranks the value of each attribute by segment and overall, and is helpful to allocate features to bundles and add-ons in the design of optimal pricing. For example, a feature with higher importance for enterprise customers could be placed in a premium package.
Willingness to pay (WTP) is an estimate of how much customers will pay for a change in the level of an attribute relative to a base feature set. It can be used to calculate a dollar value for every level of every attribute. Although WTP values are not additive, they provide a relative value for each feature versus others. Further, segmentation can be applied to WTP calculations showing variations in feature value between segments. WTP data can be used to place features in different packages. To illustrate, high value features could be included in a core offering. Willingness to pay also indicates the differences in perceived value of add-on features.
The market simulator is the most valuable output of a conjoint analysis. Attribute importance and willingness to pay can be used to develop a first-pass pricing model, but final optimization utilizes the market simulator. The inputs to a market simulator are multiple hypothetical products defined by specific levels of each attribute. Products can be specified for your company and competitors. For B2B SaaS, various scenarios are developed and tested to optimize bundles, feature placement and price levels. With all of the products specified for a given scenario, the output of the conjoint simulator is the share of choice for these products. By assuming the size of the overall market, the total revenue and estimated market share for your company can be calculated for each scenario. If cost data is added, profit can also be computed. By testing various hypothetical product configurations across multiple scenarios, you can optimize revenue or profit.
How to Get Started
The best way to get started is to assign a project leader with expertise in both B2B SaaS pricing and conjoint analysis. This leader can be an internal employee or an external consultant. Since the optimal process flow is to identify the top choice pricing model prior to the conjoint, the first order of business is to choose this pricing model. Ideally the project leader will have a proven process to develop multiple options for your pricing model. The viable pricing model options can be presented to a pricing committee and the top candidate pricing model can be chosen. An ideal pricing committee includes the CEO and representation from product, marketing, sales, customer success, finance and operations.
With the most likely pricing model in hand, your consultant or internal pricing lead can bring a market research firm with conjoint expertise into the project. The project leader then collaborates with the market research firm to develop and execute the conjoint survey. The results of the survey and market simulation are used to choose the final pricing model and bundling, define all SKUs, and set the price level for each SKU.
Conclusion
In the complex world of B2B SaaS where a diverse range of features can be priced and packaged in countless configurations, conjoint analysis is the best market research technique for pricing optimization. While the other research methodologies can only estimate pricing for a single product, conjoint can evaluate an unlimited number of product combinations and pricing scenarios to find the optimal pricing strategy. Survey data can be used to consider important segmentation in the development of bundles and add-ons. If feature tiers are being created, the market simulator can be used to optimize feature placement among the tiers. Lastly, the price level for every SKU can be chosen to optimize revenue or profit in a competitive context.
With pricing decisions that can create millions or tens of millions of dollars in value for your B2B SaaS company, conjoint analysis provides data-driven confidence that you are making the right choices.
Comments